Looking at all the stuff surrounding Lin Kayser in his Dubai office, it’s easy to assume he’s a rocket scientist working on a spaceship to escape Earth’s gravity. Drawings, plans, and prototypes seemingly stolen from an interstellar alien probe frame Kayser on Zoom. But the cofounder of Leap 71 is no rocket scientist—even if he looks like he could play one in a movie.
“We are fundamentally a software company,” Kayser tells me. “We’re building a computational model that can autonomously generate technical objects and machines.” Leap 71 doesn’t use generative AI, the predictive-based engines that power tools like ChatGPT, or even CAD software from Autodesk, nTopology, or Divergent 3D. It is not parametric CAD either. Rather, the company has developed something entirely different, a major leap in computational engineering design. Its name is Noyron, a system that is trying to harness the knowledge and creativity of engineers into a powerful artificial intelligence tool that autonomously designs advanced machinery and products.
Developed in-house, the software encapsulates the expertise of skilled engineers, integrating physics, such as thermal models, rules about manufacturing, and the logic of different engineering fields within one coherent framework. According to Kayser, Noyron is constantly evolving, learning from data fed back from real-world tests and manufacturing outcomes. The system can be specialized and tailored to serve different industries. There’s Noyron RP for rocket motors, for example, Noyron EA for electromagnetic systems, and Noyron HX for heat exchangers.
One of the defining features of Noyron, according to Kayser, is that it doesn’t just produce a shape—it predicts how that object will perform in real-life conditions. Like a human engineer, the system tries to create components that meet the desired requirements, based on the collective knowledge embedded within it. “In theory, parametric CAD should have been that, but parametric CAD is geometry-driven,” he tells me. “Our stuff is physics, it’s logic, it’s decisions, it’s iteration.”
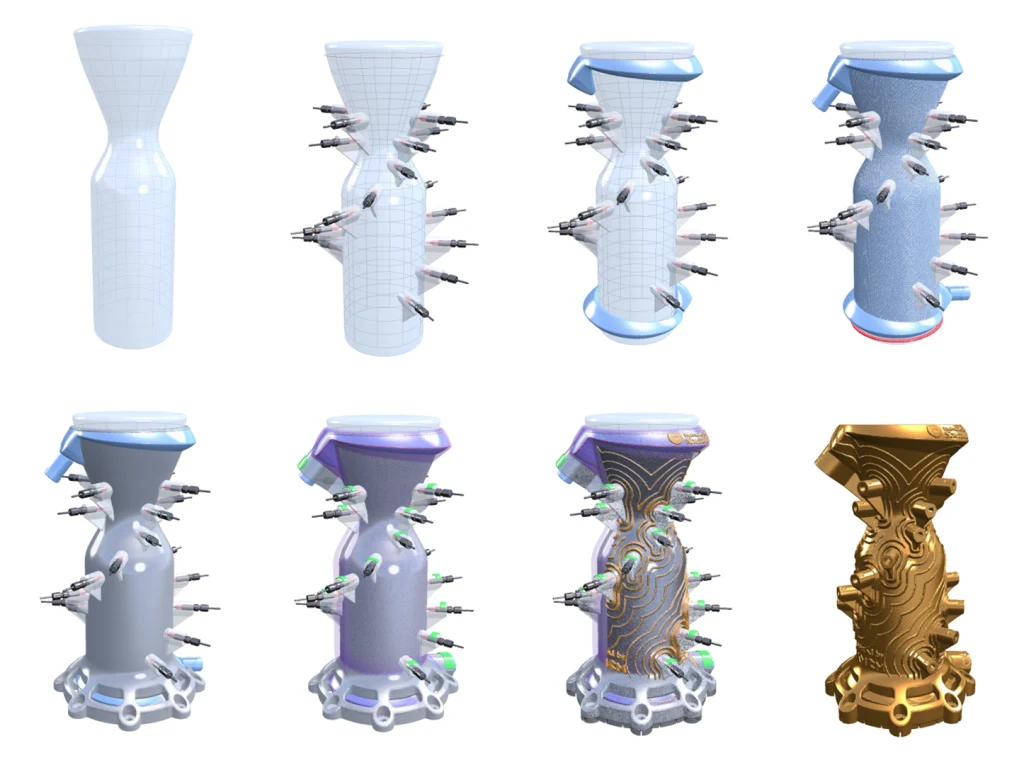
Kayser says that Noryon uses machine learning so the software knows how to think like an engineer to solve new problems. Unlike parametric CAD, which adjusts geometry, Noyron knows how to design a new record engine from scratch to match a series of specs like thrust or thermal limits. “You can’t put a thermal model in parametric CAD,” Kayser tells me. “[Noyron] is way more sophisticated than that. Calling it parametric CAD would be like saying ChatGPT is autocomplete.”
Each time a new piece of information—whether a successful test or a failure—is introduced, Noyron fine-tunes itself, getting smarter and more accurate with each iteration. Noyron doesn’t stop at generating the geometry of an object; it also outputs manufacturing files, instructions for post-processing, and physical data that can be used in simulations. It’s basically a software created not just to design objects, but to make stuff and make those objects work.
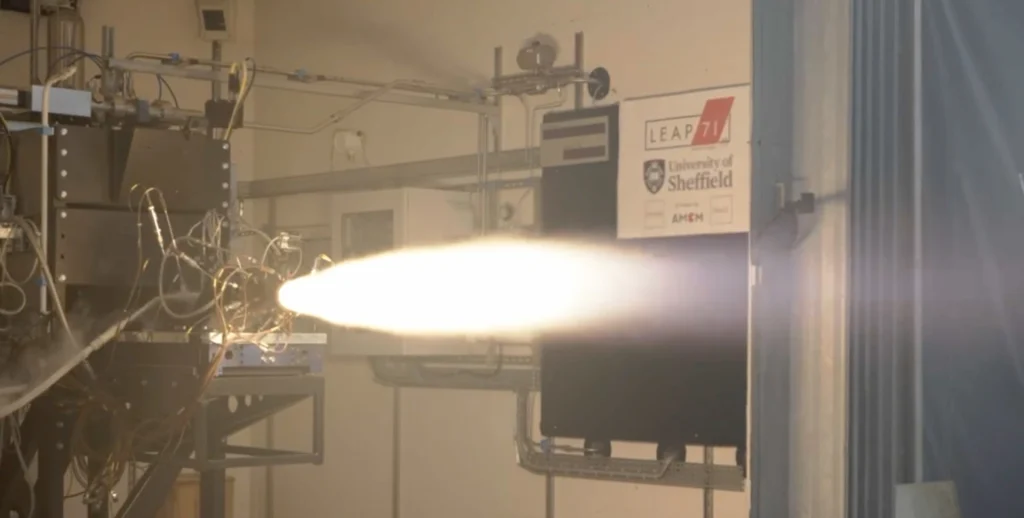
Trial by fire
Recently, the company put Noyron to the test with a 5-kilonewton rocket engine designed entirely by its computational model—the first of its kind in the world. Despite what Noyron knows and can predict, Kayser says when it comes to rocket engineering, “until you test it, it’s all theory.”
To validate the software capabilities, Leap 71, which is headquartered in the United Arab Emirates, collaborated with the University of Sheffield and AMCM, a leading European 3D printing firm, to build and test the engine. The engine runs on liquid oxygen and kerosene—an inherently challenging combination due to their volatility and the extreme conditions they require. “Liquid oxygen is extremely reactive,” Kayser says. “Even a small piece of copper in the wrong place could cause a spark and lead to an explosion.”
The team installed the engine in an old WWII-era bunker in the U.K., retrofitted with modern aerospace testing capabilities. Old concrete walls sheltered the alien-looking engine from conditions and materials that could cause a reaction.
The first test burn lasted just three seconds, with fire bursting out of the exhaust nozzle. It worked. But there was no immediate celebration, Kayser says, only a silent, collective acknowledgment that they were on the right path. Then they tried a second, longer burn of 13 seconds. This one confirmed the engine’s stability and reliability. The software had actually made a rocket engine that worked as predicted.
Kayser tells me that the engine worked after printing directly from the CAD file because of this technology. Moving traditional engineering knowledge into a computational space allows AI to achieve in minutes what would take a skilled human engineer weeks or even months. It’s not just feeding a large language model a ton of books on rocket engine design. It’s about carefully setting rules while learning the knowledge of rocket engineering. The successful test shows that this may be a fundamental reimagining of the engineering process—one in which AI serves as a true partner rather than just a tool.
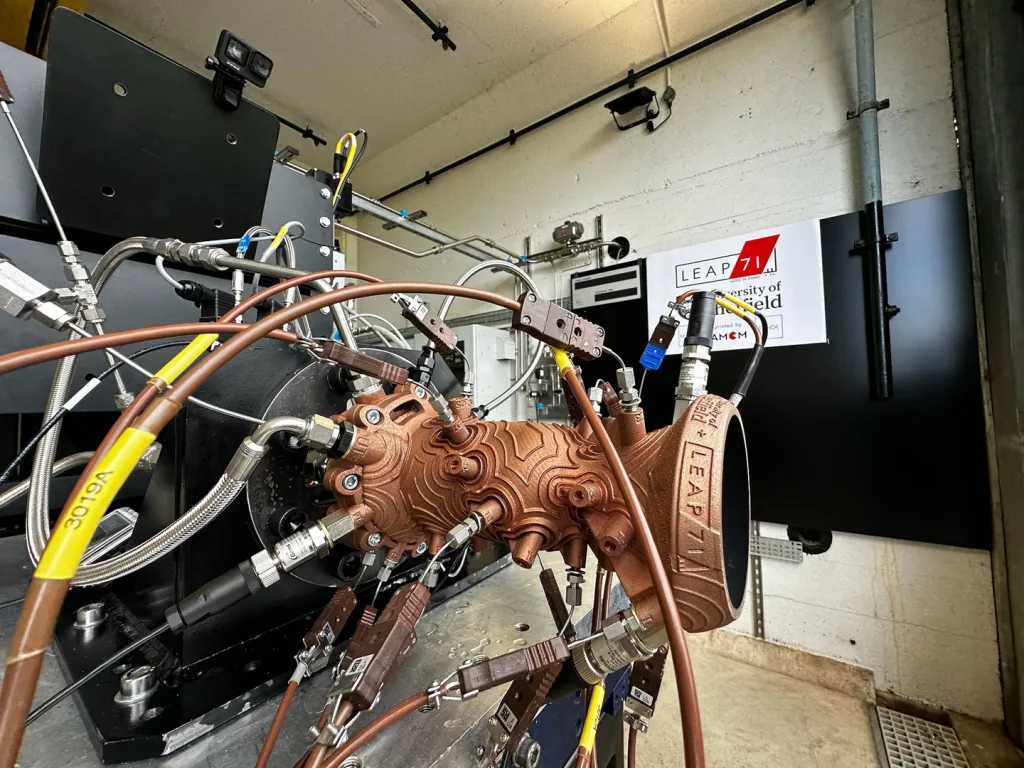
Consistent output
Noyron operates differently from generative AI solutions for modern CAD software, Kayser says. These can be unpredictable and produce results that vary with each run. They require an engineer to supervise the resulting parts generated by the AI, which has to use its knowledge and intuition to spot what’s wrong and fix it, one prompt at a time. Just like when you use ChatGPT or Gemini.
To understand the difference between Noyron and current CAD software generative AI tools, look no further than the work coming out of NASA. Ryan McClelland, a research engineer at NASA’s Goddard Space Flight Center, has been working on using generative AI to design spacecraft components to increase strength while shaving precious weight. His strange-looking “evolved structures,” as he calls them, are used in complex mission hardware, from parts in astrophysics balloon observatories to future planetary probes’ instruments.
McClelland explained to me that generative AI design starts with a prompt, similar to how people use it to generate images, text, or music. In this case, the prompts include physical requirements—geometric interfaces, specific loads, and conditions, like having to sustain 60 g-force acceleration. The AI then creates a part that meets these specifications, iterating dozens of designs within hours. While this speeds up the design process, it still requires human oversight.
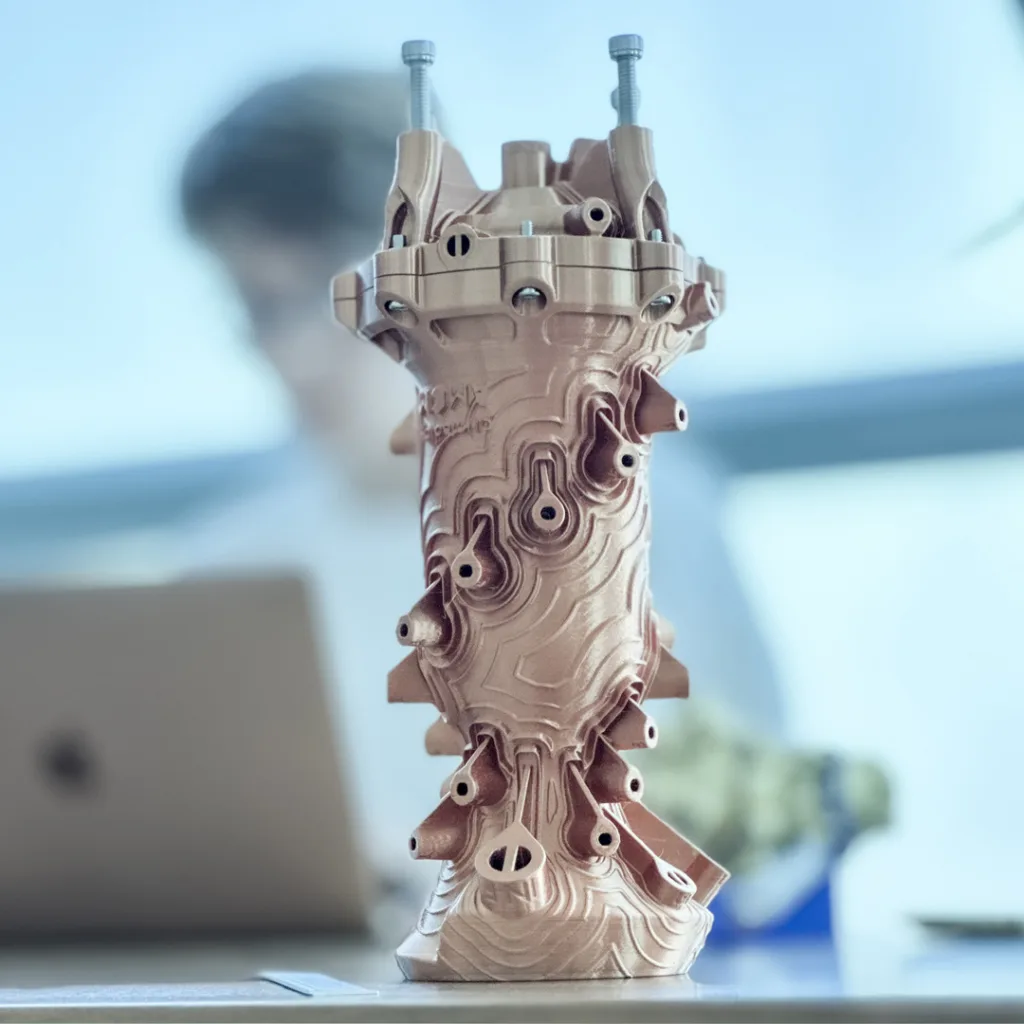
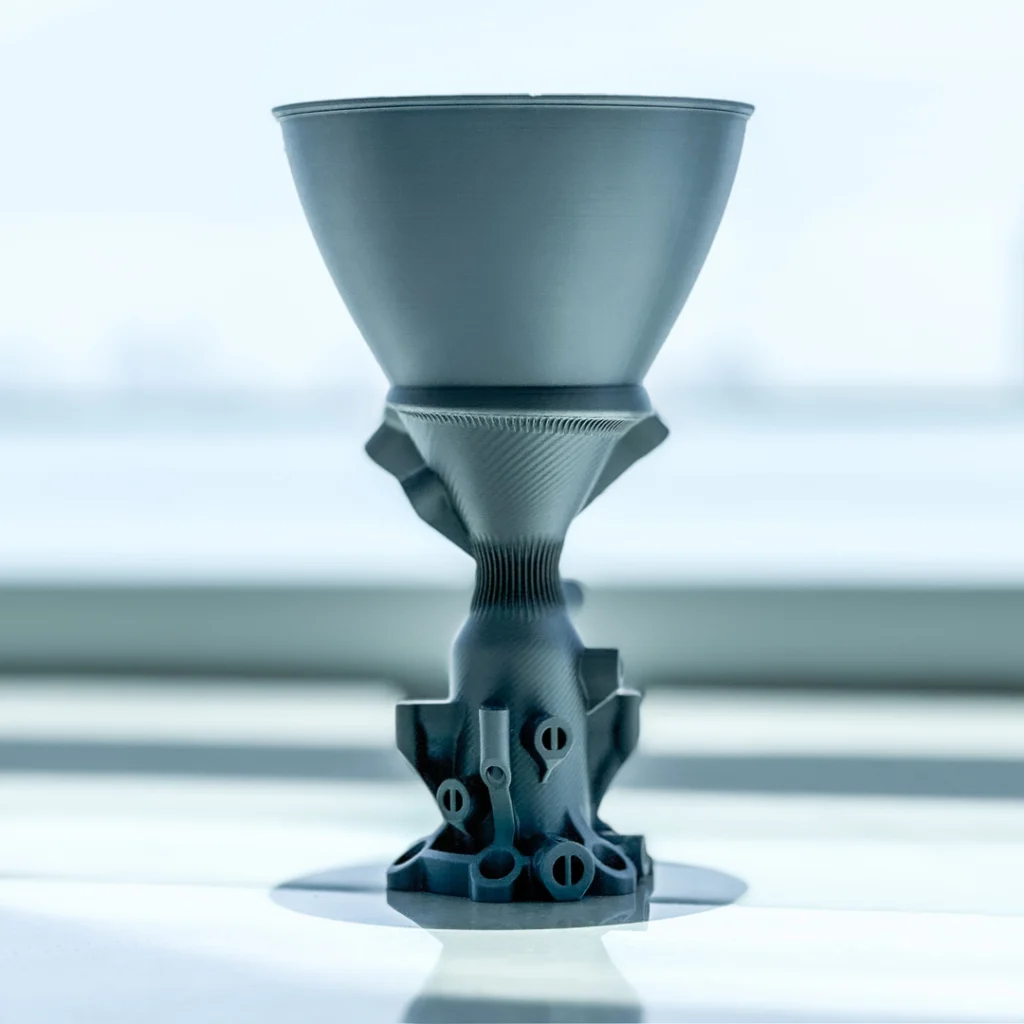
The AI’s suggestions may include impractical features, such as parts that are too thin or not structurally sound for the intended purpose. “You still have to apply human intuition,” McClelland notes. While generative AI can quickly produce multiple designs, he says, the process cannot move forward without a human engineer’s intuition and understanding of practical limits.
Whereas generative AI acts more like a helper to the engineer, offering possible designs that humans must then validate and refine, Noyron aims to produce end-to-end work. Kayser likens the software to an “engineer-brain-in-a-box,” where rationale is given for every decision the computer makes. This is essential in the context of aerospace engineering. Designing rocket engines is an unforgiving task; every detail must be accounted for, and mistakes can be catastrophic. Unlike the black-box nature of generative AI models, where decisions may be opaque, Noyron’s approach is transparent, making it easier for engineers to understand why certain design decisions are made.
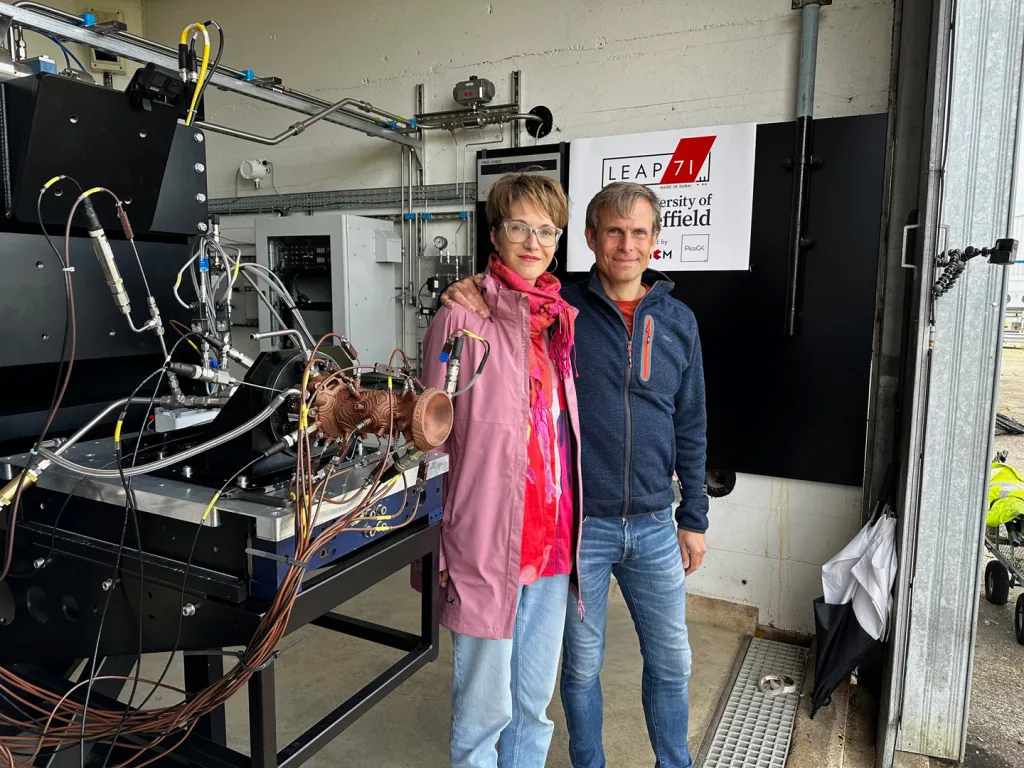
The importance of human know-how
The transparency of Noyron makes it especially effective for human-machine collaboration. Engineers using Noyron can see the rationale behind each design decision, which makes it easy to adjust the model’s logic or tweak inputs to improve outcomes. Nothing is arbitrary. Rapid iteration is key here too, Kayser says, as Leap 71 software can refine designs to meet the practical requirements of aerospace systems much faster than with traditional approaches. The model can iterate on designs in minutes, allowing engineers to explore different configurations and select the optimal solution without having to start from scratch each time. But given a set of specs (you can call it a prompt), the result will always be the same. This ensures the reliability needed in such a critical field.
Kayser believes that future versions of Noyron could bring Moore’s law to engineering—which refers to the observation made by Gordon Moore, cofounder of Intel, that the number of transistors on a microchip doubles approximately every two years, leading to the same boost in computing power while costs decrease. This principle has driven the rapid growth of technology for decades, resulting in exponential progress in computing capability.
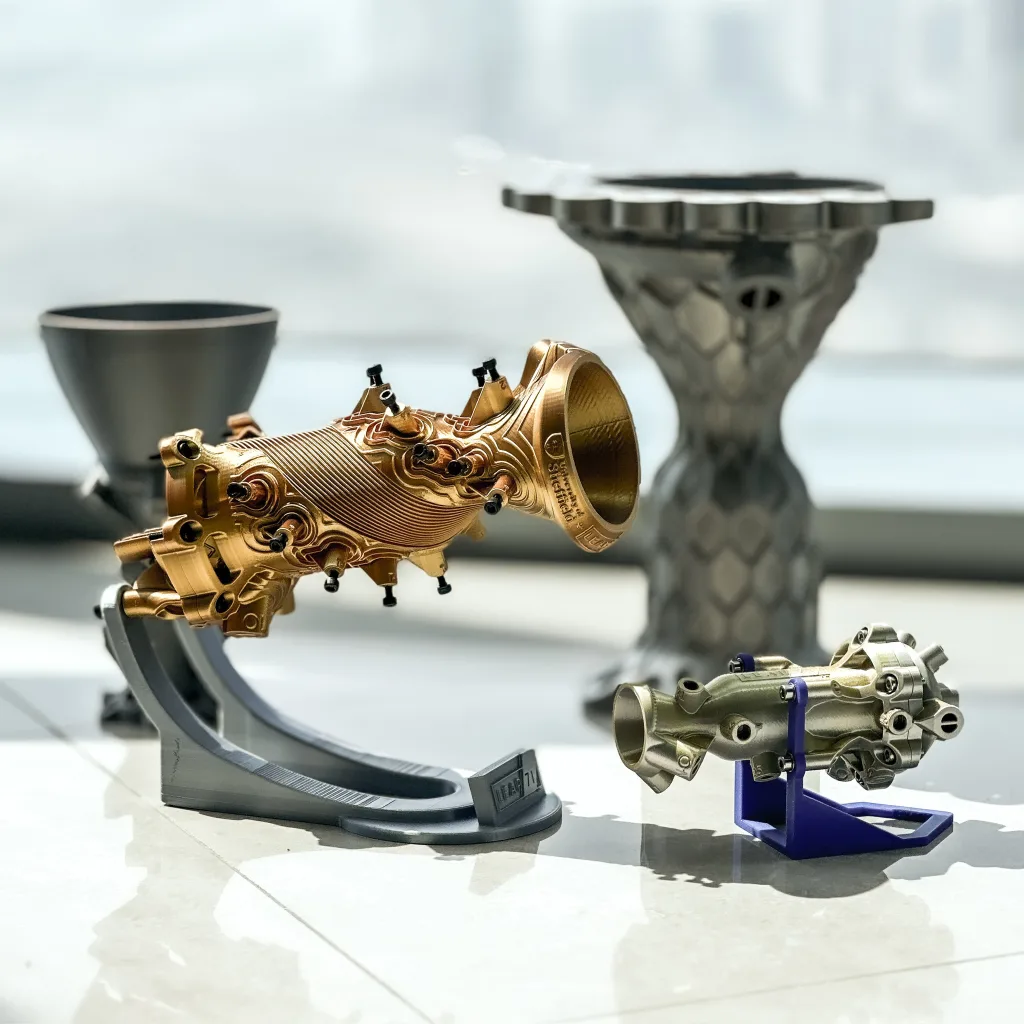
“It’s about making engineering computational and applying the principles of software development to physical object design,” Kayser says. Instead of the slow, painstaking process of drafting designs, engineers can use Noyron to instantly explore different possibilities—such as swapping materials or adjusting design parameters—and receive immediate feedback. This capability allows for a level of creativity and risk-taking that was previously impossible. Engineers no longer have to be afraid of the time cost associated with experimenting. What used to take months can now happen in minutes, and that changes everything.
Kayser says companies customize Noyron based on their own knowledge, experiences, and specific requirements. “Companies don’t just use Noyron off the shelf,” he tells me. “They add their own proprietary knowledge, their way of approaching problems, into the system. Noyron gets smarter and tailored to reflect the specific expertise of the company that’s using it. It’s like having an engineering team that already knows your unique methods and priorities.”
That allows human engineers to build their unique expertise into the system, which results in new engineering solutions that reflect the company’s particular approach. The ability to integrate diverse sets of experiences into Noyron means that different companies can come up with different solutions even when starting from the same specifications.
By encoding engineering expertise into a computational model, Kayser says, Leap 71 is creating a shared language that engineers from various disciplines can use for collaboration. “Engineering is often fragmented and relies on the knowledge of individual experts,” he says, noting that Noyron aims to unify this scattered expertise, providing a reusable and adaptable model that engineers can use to collaborate across disciplines.
Another major strength is Noyron’s ability to integrate real-world test data directly into future designs. Traditional engineering methods keep design and testing phases separate, making the iteration process slow. Each cycle of testing and redesign can take weeks or even months. Noyron changes this by allowing data from each test—whether the design succeeds or fails—to feed back into the model seamlessly. “Innovation depends on iteration,” Kayser says. “There’s no way you can just come up with a perfect design in isolation; you have to test it.”
This feedback loop not only makes Noyron smarter but also accelerates the entire engineering process. Instead of waiting weeks for test results to inform design adjustments, engineers can see the impact of test data in real time and make necessary changes immediately.
Making the real-life Tony Stark’s Jarvis
Leap 71’s ambitions go beyond rocket engines. Kayser hints that they are working toward building the first general-purpose AI for engineering—a system capable of designing anything from rocket components and heat exchangers to a fuselage, dam, and fusion engine in the extremely far future.
“It sounds like you’re trying to create something similar to Jarvis, the AI that designs stuff for Tony Stark in Iron Man—an AI that can do pretty much anything,” I say, surprised by his ambition. “Exactly,” he says. “We want to build the first general-purpose AI for engineering, something that can be applied to a wide range of problems, not just aerospace.”
Imagine having an AI assistant that knows all the details of your company, your industry, and can come up with solutions almost like a team of engineers, but faster. The successful test of the 5-kilonewton rocket engine is just the start, a proof of concept that demonstrates Noyron’s potential across a range of applications. “We’re aiming for something that can revolutionize not just aerospace, but anything that needs complex engineering,” Kayser says.
He imagines a future in which computational models like Noyron are ubiquitous across industries—to design everything from cars to consumer electronics, fundamentally altering how products are engineered. The timeline, of course, may be uncertain, but early results like this rocket engine and others that Leap 71 and its partners have achieved are promising. The Noyron models initially developed for aerospace are already being adapted for other uses. The thermal models used in rockets, for instance, are finding applications in HVAC systems. This kind of cross-pollination, Kayser claims, shows the multi-industry path ahead.
The path to a future in which engineering innovation is accelerated to this degree is a long one, for sure. But Leap 71’s idea may actually work, at least judging from its rocket test. It’s a great first step to transform engineering from a manual, slow-paced process into a computational solution that is agile, inherently collaborative, and accumulates knowledge to speed up creation of new wares. If Kayser’s company—or anyone else with a similar approach—can pull off this Jarvis idea, it will be a fundamental shift in how humanity’s future will be designed and built. That cool 5-kilonewton rocket engine is a great technological achievement, a world first, yes. But more importantly, it’s a glimpse into a new era of design.